Section 3: The Innovation Sweet Spots approach
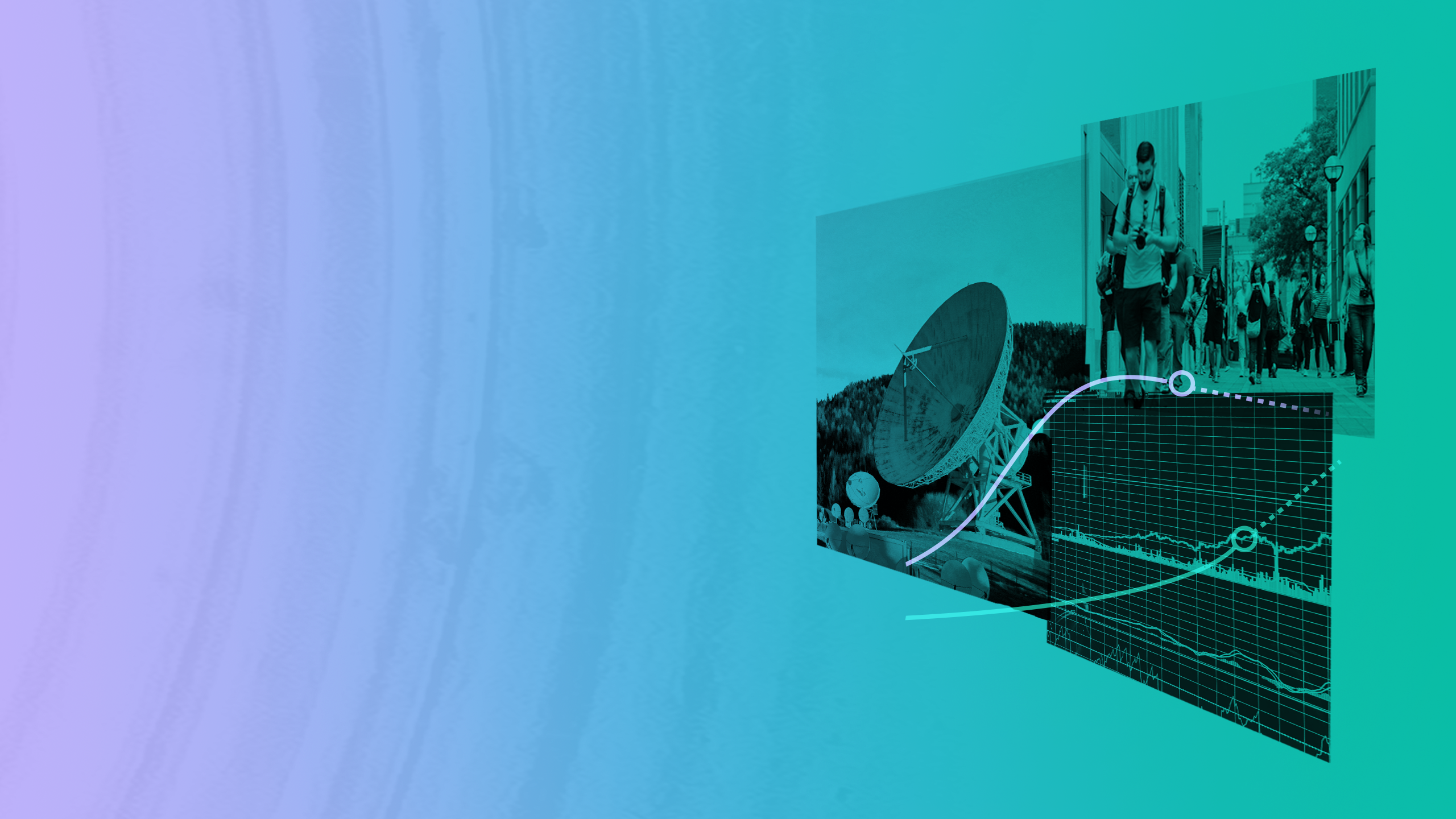
This section provides an overview of our data-driven horizon-scanning approach, as well as offering recommendations on strengthening insight into the UK’s innovation system. It will be most relevant to readers such as innovation policymakers, researchers, strategists and foresight practitioners.
In forthcoming articles we will describe the methods in greater detail, together with making the accompanying analysis code available publicly.
Innovation Sweet Spots analyses multiple signals about innovations to explore the resources they are attracting and how they are perceived. We use historical data on research activity and funding, investment into private businesses, news media coverage and parliamentary debates. Our overarching aim is to detect and contextualise present-day trends around innovations so that we can extrapolate potential futures ahead.
This multi-dimensional approach reflects the fact that socio-technical transitions (such as the decarbonisation challenge) are influenced by a multitude of factors. They range from research and development of new technologies, to policy, business strategies, social acceptance and cultural discourses.
Identifying tipping points – critical thresholds where a relatively small intervention can trigger an outsized larger change – could help us to facilitate major societal shifts such as the post-carbon transition. This is similar to the idea of critical mass, where a process such as adoption of new innovations or formation of a public opinion reaches an inflection point and becomes self-sustained.
To identify technologies or innovations that might be at such a tipping point – a sweet spot – we’re looking for a high level of research activity and strong growth in private investment alongside an escalating degree of interest from policymakers and the public. Taken together, signals of this nature would be indicative of a maturing ecosystem underpinning an innovation that is capable of cutting into the mainstream. These would be the preconditions for any innovation to make a significant impact on a social challenge.
In this pilot, we have tested an approach that uses data science and machine learning methods for exploration and characterisation of technologies and innovations.
Datasets and methods for capturing signals
Our choice of datasets reflects our aim of capturing different elements of the diffusion of innovations framework set out by Everett Rogers, namely the innovation and innovators, the media and the social system.
To capture research trends, we used the open-access Gateway to Research database. It contains information about projects supported by UKRI, which is responsible for funding a large proportion of UK research and innovation (£5.3 billion or almost 39 percent of the total R&D expenditure in 2019). Relevant to the green technology focus of this pilot, UKRI has a large portfolio of projects supporting the net-zero goal. These projects might be carried out by public as well as private organisations, and include both more basic and more applied research. Importantly, this type of data has the advantage of being an early signal compared to publications or patents, as the project abstracts describe research plans for several years ahead. Within this data, we identified research related to specific innovations and examined the trends in the number of projects and magnitude of funding.
To examine investments into businesses, we used the Crunchbase database, which contains information about the amount of money raised by companies from investment deals, ie, investment rounds, grants, seed funding and more. While Crunchbase is a global company directory, we restricted the scope of this initial pilot to the UK companies in the dataset.
To identify technologies in the research and business investment data, we analysed the research project abstracts and business descriptions using unsupervised machine learning methods as well as simpler keyword searches. We leveraged topic modelling (namely, top2vec and latent Dirichlet allocation methods) to explore the datasets and automatically identify projects and businesses related to green technologies and heating. Based on this, we tailored a set of specific search keywords and phrases to refine the automated results and achieve a satisfactory level of granularity for our technology categories.
Where possible, we also used the existing research project and business labels specified in the Gateway to Research and Crunchbase datasets (note, however, that these datasets do not feature labels specific to heating technologies). Finally, we manually reviewed all low carbon heating and a subset of other green technology research projects and businesses to remove false positives.
We complemented the insights about research and investment with public discourse analysis to better understand how technologies are being talked about in the public arena. We used openly available records of parliamentary debates (Hansard) and the open access platform of The Guardian news website, which, to the best of our knowledge, is the only major UK newspaper to make its text freely available for research.
To detect news articles and speeches in the House of Commons that mention specific technologies, we leveraged the tailored set of search keywords and phrases. For select technologies (heat pumps and hydrogen heating) we carried out more detailed content analysis to remove false positives, extract sentences mentioning the technology terms, and automatically detect other important words and phrases that are often co-located with the technology terms.
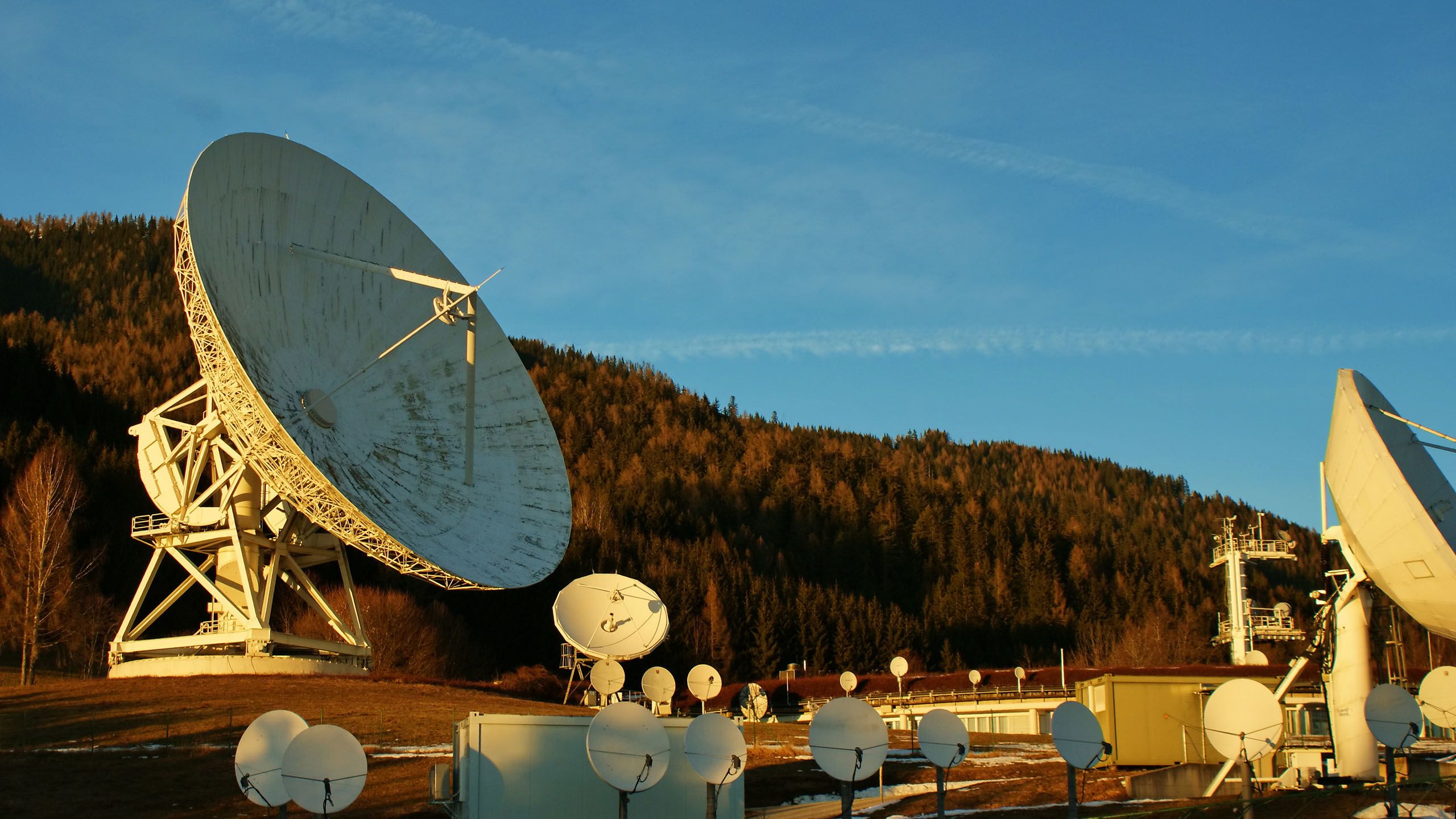
Making sense of trends
To interpret and communicate the trends around research, investment and public discourse, we prototyped a data-driven typology that categorises these trends as dormant, emerging, hot or stabilising (Figure 1).
Our typology takes into account the magnitude and growth of time series related to a particular technology, such as the number of new research projects or the amount of investment across different years. Growth is one of the main attributes when evaluating emerging technologies, whereas magnitude can help us understand and compare where the innovations and technologies are in their life cycle. We focused on five-year trends throughout this study, and we generally report trends up until 2020 (unless noted otherwise) as data for 2021 was incomplete.
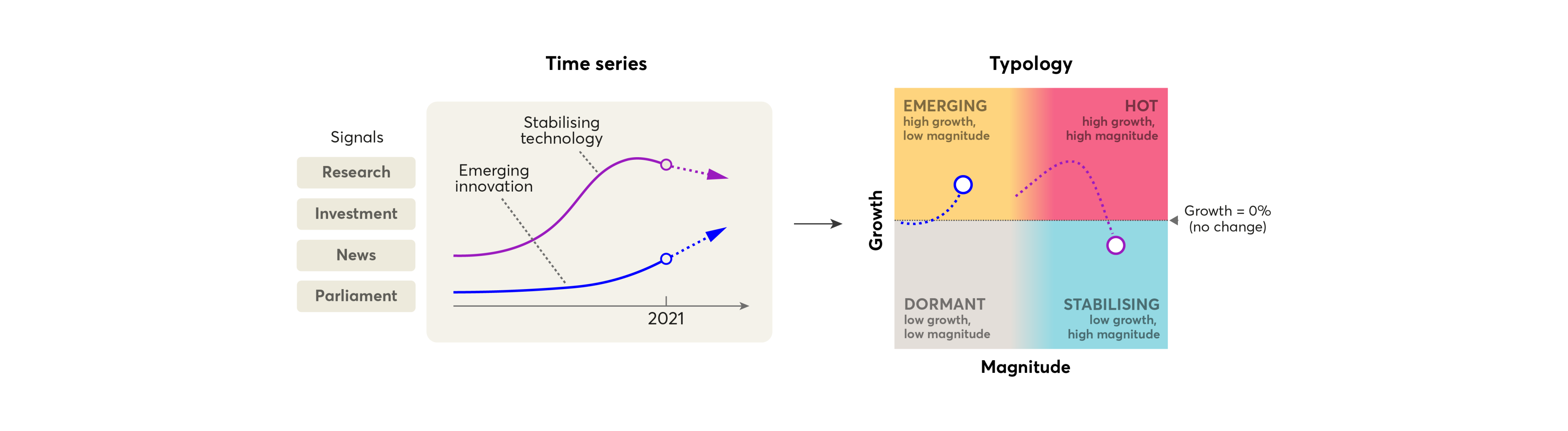
Figure 1. Typology of time series trends. Magnitude is calculated as the average value of the signal (e.g. amount of research funding) across a five-year period, whereas growth is the percentage increase of the (smoothed) signal value in the same time period.
Figure 1. Typology of time series trends. Magnitude is calculated as the average value of the signal (e.g. amount of research funding) across a five-year period, whereas growth is the percentage increase of the (smoothed) signal value in the same time period.
To smoothen the year-to-year fluctuations of time series, we calculated the magnitude as the average value across the past five years (between 2016 and 2020). Growth was estimated by calculating a three-year rolling mean (up to 2020) and then calculating the percentage increase of the smoothed values at a given year compared to four years ago. For example, the growth for the year 2020 will compare the mean across 2018 and 2020 versus the mean across 2014 and 2016. In this way, the growth percentage estimate captures a smoothed growth trend across the past five years.
We categorised signals with a positive growth percentage as either emerging (positive growth but relatively low magnitude) or hot (positive growth and large magnitude). Conversely, signals with a negative growth percentage were deemed as stabilising (negative growth but large magnitude) or dormant (negative growth and small magnitude).
Our hypothesis (which would need to be validated through further iterations of Innovation Sweet Spots) is that technologies will likely transition through each of these categories depending on the life cycle stage of the technology and the associated products. The rate of growth can be expected to typically diminish as the volume of activity grows (in line with the so-called S-curve pattern in innovation). In this context, outliers in the hot area of the typology will be particularly intriguing.
We make the distinction between emerging and hot, and between dormant and stabilising, in relative terms by comparing the technologies pertaining to the social challenge domain and drawing the threshold at the median magnitude value. We appreciate, however, that there is no hard objective threshold between these categories and there is also an element of qualitative judgment when using this categorisation to summarise trends across multiple signals (eg, the number of research projects and the amount of research funding).
In summary, by applying our typology to score the magnitude and growth of signals from the various different sources and assessing them all together, we aim to paint a multi-dimensional picture of the activity around innovations.
Limitations
We trialled a mixed-methods approach for Innovation Sweet Spots. Findings from the data analysis were cross-referenced with qualitative feedback from experts at two stages. We sense-checked our findings with a range of specialists in emerging technologies, energy and environment, policy and venture capital. However, we want to highlight some of the limitations of our methodology.
Firstly, there are gaps in our data in terms of the coverage of public and private research and development spending. Hence, the funding and investment amounts that we report should be seen as ballpark estimates. Funding for technologies could come from other sources besides the ones listed in Gateway to Research database, such as internal company investments, direct grants from government departments or funding from European Union programmes.
Moreover, a single project can include several technologies (eg, community heating projects combining different solutions) and hence not all of the project funding amount will be directed to one specific type of technology. We aim to increase the coverage of funding sources as we develop Innovation Sweet Spots further.
The findings based on the Crunchbase dataset are limited by the fact that raised investment data has been reported only for 23 percent of companies that we identified through our research (which is, however, higher than the Crunchbase average for UK companies of 11 percent). This might be due to a variety of reasons, such as Crunchbase’s reliance on crowdsourcing for data collection and the fact that some sources of funding are not publicly disclosed.
The development of certain technologies might be dominated by large, established companies and less probable to attract venture capital funding, therefore making it less likely to be picked up in the Crunchbase dataset. It is also important to note that business description texts which are often rather concise might not reflect the full extent of the company’s activities.
Finally, the semi-automated nature of our analysis approach can result in occasional false positive or false negative results, and we will continue working on a rigorous evaluation of the level of uncertainty in our estimates.
Therefore, we encourage you to interpret the results of this work as data-informed hypotheses about research and innovation trends in this space – as opposed to a comprehensive analysis. We hope these insights nonetheless make a useful contribution and prompt further investigation about where best to invest in this critical field of innovation.
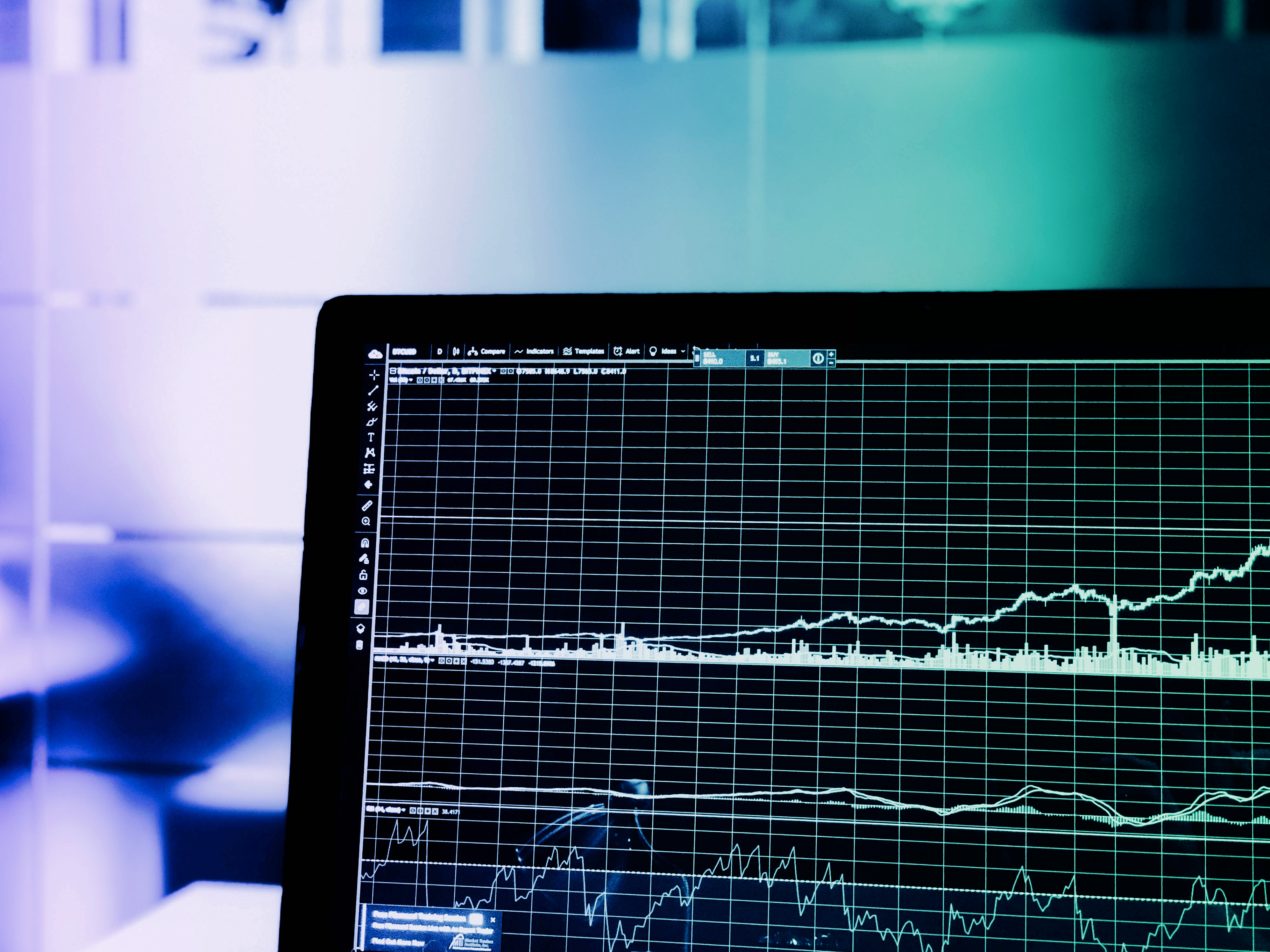
What’s next for Innovation Sweet Spots?
This pilot is the first step in the Discovery Hub’s approach to data-driven horizon scanning on innovation trends. Going forward, we’d like to develop our approach further into a general purpose methodology and infrastructure to characterise emerging technologies and innovations being used to tackle a range of different social challenges across domains such as health or education.
Besides the overall trends that we are studying in this article, the datasets we have used in our pilot open the door to further interesting questions. For example, by using the geographical and institutional metadata of the research projects, one can identify public and private organisations as well as locations in the UK that are particularly innovative in specific technologies. Moreover, it is possible to analyse interactions between organisations to understand the development and structure of research collaboration networks (for example, how centralised or decentralised they are). Nesta has previously carried out a similar type of analysis in the context of UK creative industries and Welsh research organisations.
Another promising prospect is the scope to move from retrospectively tracking trends to forecasting future trends and anticipating change by using quantitative as well as qualitative and participatory methods. In this context, research project data, being an early signal, provides a window to future trends in innovation. A more detailed analysis of the research project descriptions could be combined with the insights from trends analysis, and used to support foresight exercises. For example, by extrapolating from existing trends it would be possible to create evidence-led scenarios to help develop strategic responses to different futures (Figure 2).
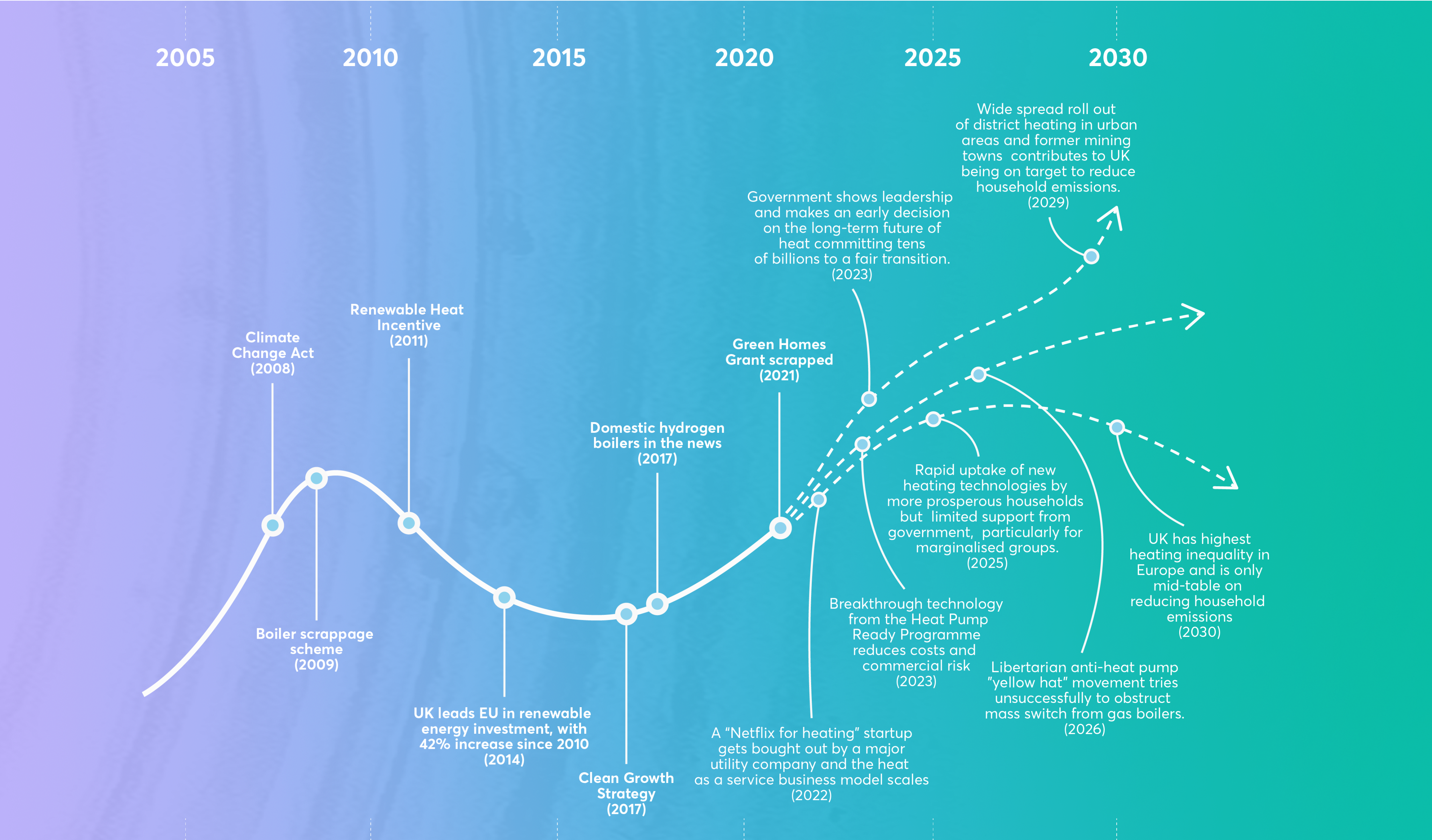
Figure 2. Examples of speculative future scenarios that could be further enhanced by using data-driven methods.
Figure 2. Examples of speculative future scenarios that could be further enhanced by using data-driven methods.
Enriching and linking innovation data
Innovation Sweet Spots and Nesta’s previous work on innovation mapping (for example, on the use of AI in creative industries) demonstrate the value of datasets like the Gateway to Research in understanding the UK innovation system. However, one of the main challenges of this pilot was identifying specific types of low-carbon heating technologies in the research project and business information datasets. Another challenge (addressed earlier in this article) is the access to internal R&D data from within companies which might help all stakeholders better understand the innovation landscape.
Public datasets related to research and business activity should be labelled more comprehensively to allow easier access to timely insights about trends relevant to social challenges.
This would benefit the government in two ways. Firstly, it would support its own internal analysis (which seems likely to become mission focused following the recent Innovation Strategy) and secondly, it would strengthen analysis undertaken by others which the government could then use to inform policymaking.
For such labelling to be most useful, it should be open-source and aligned with taxonomies used by industry, the third sector and government such as that being created by the Government Office for Science. This will require developing algorithms for automated text labelling, as well as inputs from domain experts and cross-institutional collaboration. Related to our pilot project, an example might be joining up the Energy Technology List maintained by BEIS with the relevant research projects in the Gateway to Research database.
A standardised, automated methodology to detect technologies and challenge areas within text data could also facilitate the joining up of public research funding information from sources that are not covered by the Gateway to Research database; for example, departmental funding. Moreover, business information platforms would be encouraged to adopt standardised categories of technologies or challenges, thus allowing for a better measurement of impact investment. For example, the Dealroom business information platform is already labelling companies according to the UN Sustainable Development Goals.
Over the long term, by contributing to the evidence base which underpins public investment in innovation, this would bolster the government’s efforts to enhance the UK's reputation as a world leader in the field.
Whilst it is subject to commercial sensitivities, data on company investments in R&D would provide considerable insight into trends in innovation currently hidden from view. Currently, companies have little reason to make data on their internal innovation public. A way this could be achieved in practice is through data trusts, a type of data institution that allows individuals or groups to pool resources, tasking an independent ‘trustee’ to manage those resources for the benefit of the trust’s members. Not only would unlocking comparable data across companies create public value, it could also benefit industry as they could draw on intelligence from beyond their internal teams.
Strengthening the innovation system
Innovation Sweet Spots highlights a wider opportunity to strengthen the UK innovation system by adopting data driven approaches and engaging civil society organisations that work in this field. This would enrich the evidence available to decision makers by providing insights from new sources, emphasising public benefit and offering improved understanding of the potential unintended consequences of technological innovation.
In June 2021, the prime minister announced the establishment of a new ministerial council and Office for Science and Technology Strategy (OSTS) to provide direction on how science and technology will tackle great societal challenges and transform lives. One of the first tasks of the OSTS will be to review the technology bets the UK should back and prioritise for strategic advantage. As part of this review the Ministerial Council and OSTS should consider using evidence from data-driven horizon scanning and from civil society organisations with deep knowledge of the social challenges and context for the deployment of technologies.
We plan to continue refining our methodology and exploring the predictive potential of these datasets. If you have any advice or want to discuss the project, please get in touch.
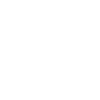