How could generative AI change early-years education?
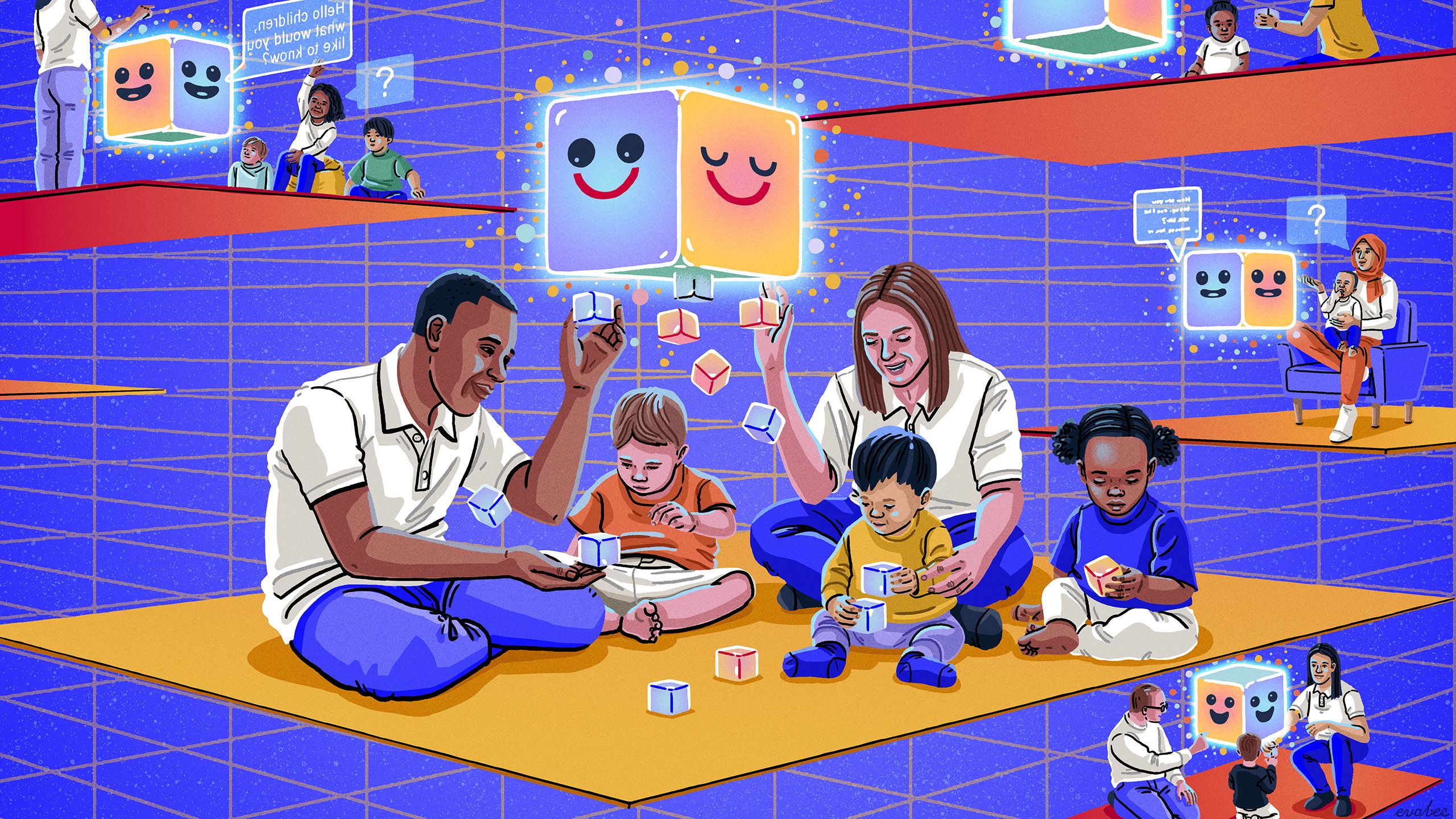
Answering tricky questions, creating engaging activities and accessing trustworthy advice are challenges that any parent or early-years practitioner will recognise. We’ve been exploring the potential for generative AI tools such as ChatGPT to be used for social good, and wanted to imagine how the technology could help early-years practitioners. Generative AI is a type of artificial intelligence that learns from existing data to create new content such as text, images or music.
Staff shortages, creative burnout and administrative overload are the biggest issues facing the early-years education system today, according to the experts we interviewed. AI has the potential to help: our conversations with AI experts identified over 40 ways generative tools could support the workforce.
We created three prototypes to help our experts reflect on the potential impact of this technology in the real world. These prototypes use large language models: AI that has been trained on huge amounts of data to generate high-quality text.
We invite you to test these prototypes, bearing in mind the following:
• these prototypes are for demonstrative purposes and should not be used by children or in early-years settings
• generative AI can make mistakes. Nesta cannot control and is therefore not responsible or liable for responses given by these prototypes
• do not submit confidential, sensitive or personal information, as Nesta cannot guarantee the security or privacy of third-party generative AI tools
• our use of government and NHS content does not imply their participation, contribution or endorsement of our prototypes.
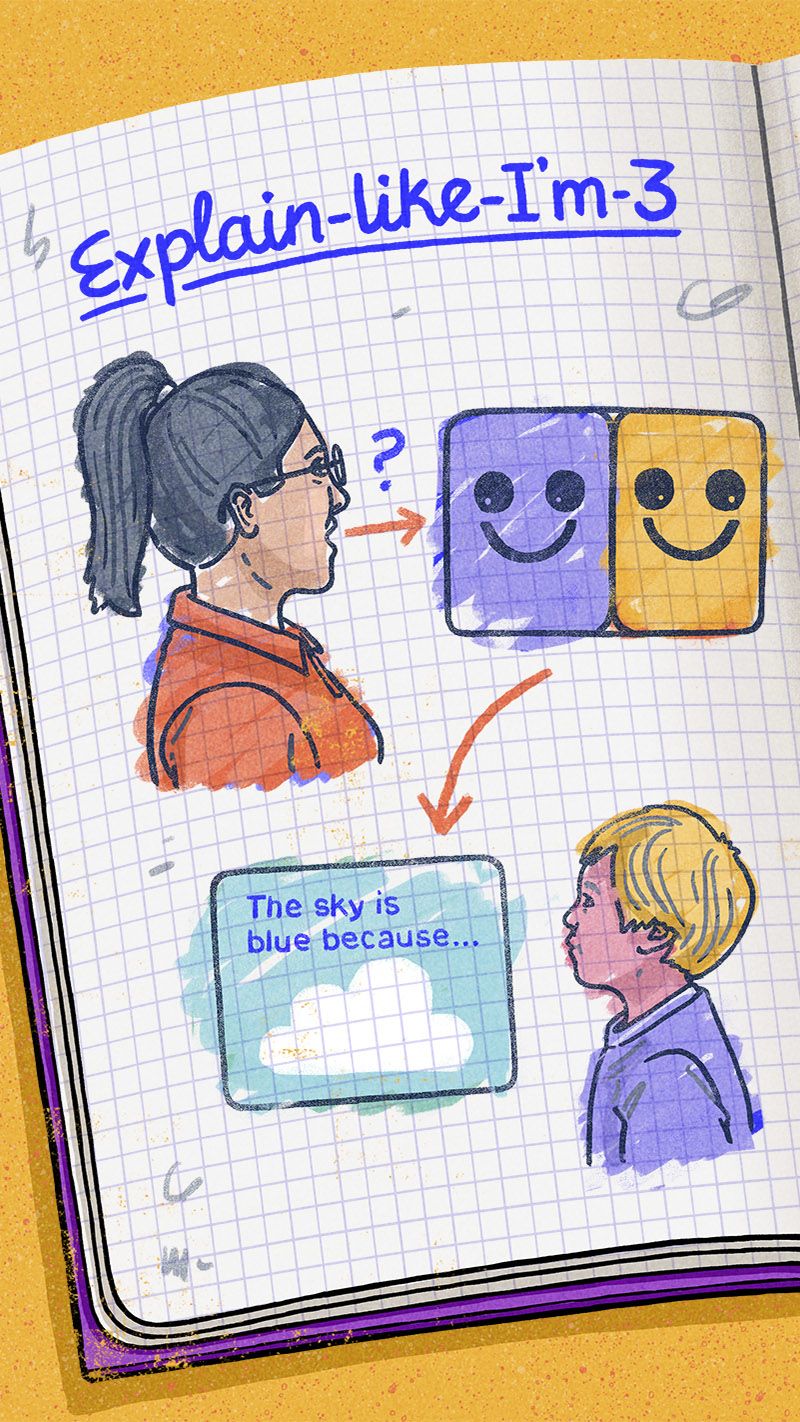
Prototype 1: Explain like I’m three
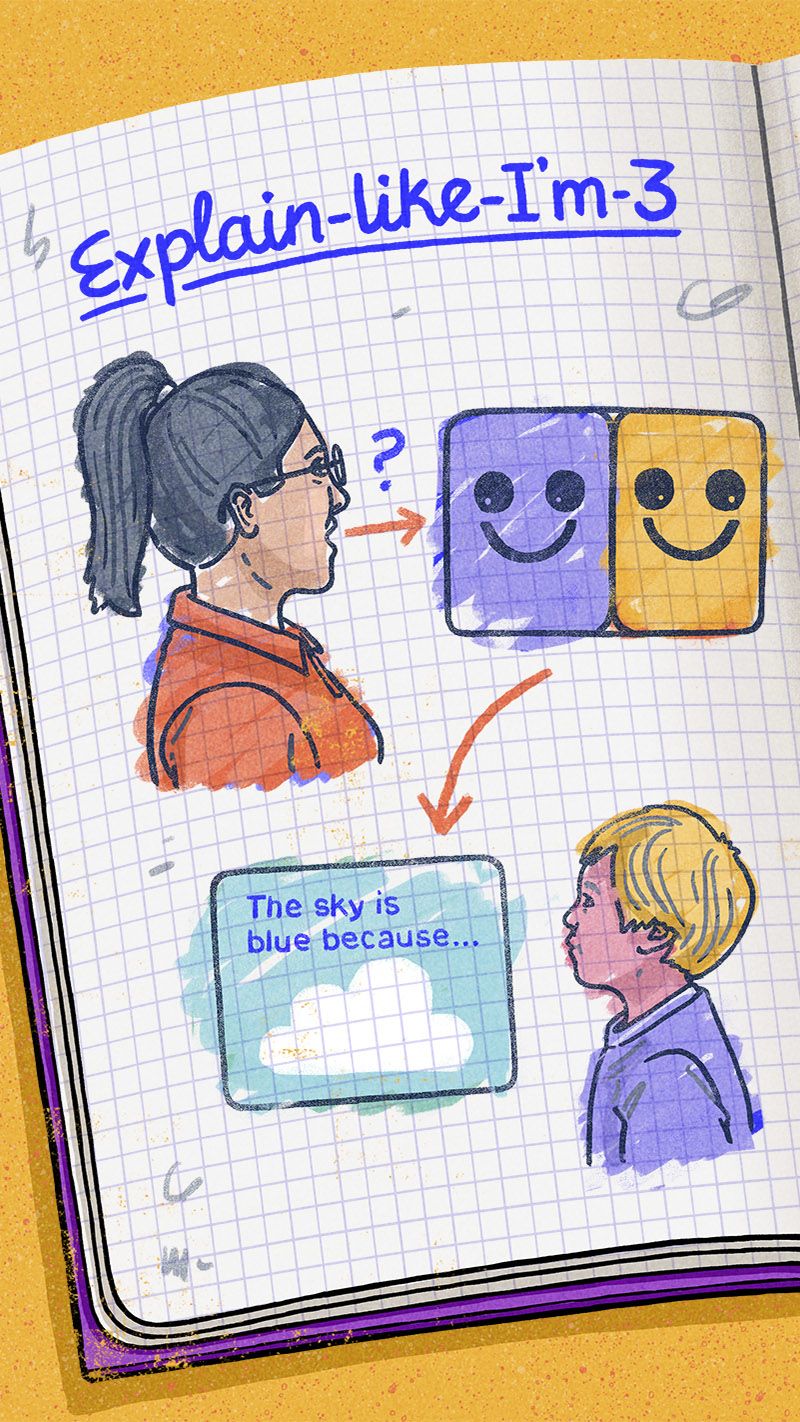
This prototype responds to questions about complex concepts with age-appropriate explanations, aiding ‘contingent talk’, the practice of responding to a child’s behaviour and questions, which is vital for language development. We also instructed the AI to avoid gendered language.
Try it out
Imagine you are an early-years educator in a nursery, working with curious three-year-olds who ask lots of questions: why does the moon change shape? What happens when we sleep? Why do people speak different languages?
Type your questions below to see a response. Note that you can also ask follow-up questions to the chatbot, such as “explain in more detail” if the first response is too simple, or “can you come up with more questions?”
Watch a video of this prototype in use.
Our experts’ verdict
- The explanations generated by the prototype use clear language and creative analogies that will help children to understand answers.
- However, responses are unpredictable, sometimes factually incorrect, and may perpetuate existing biases, which is particularly concerning given children's developing brains. That said, a future iteration of this prototype could personalise content to improve experiences for children from minoritised groups.
- Using this prototype would interrupt a conversation with a child. Engaging immediately with a child to encourage curiosity is more important than giving accurate answers to their questions. Practitioners could instead use this prototype between conversations for inspiration.
Prototype 2: Personalised activity generator
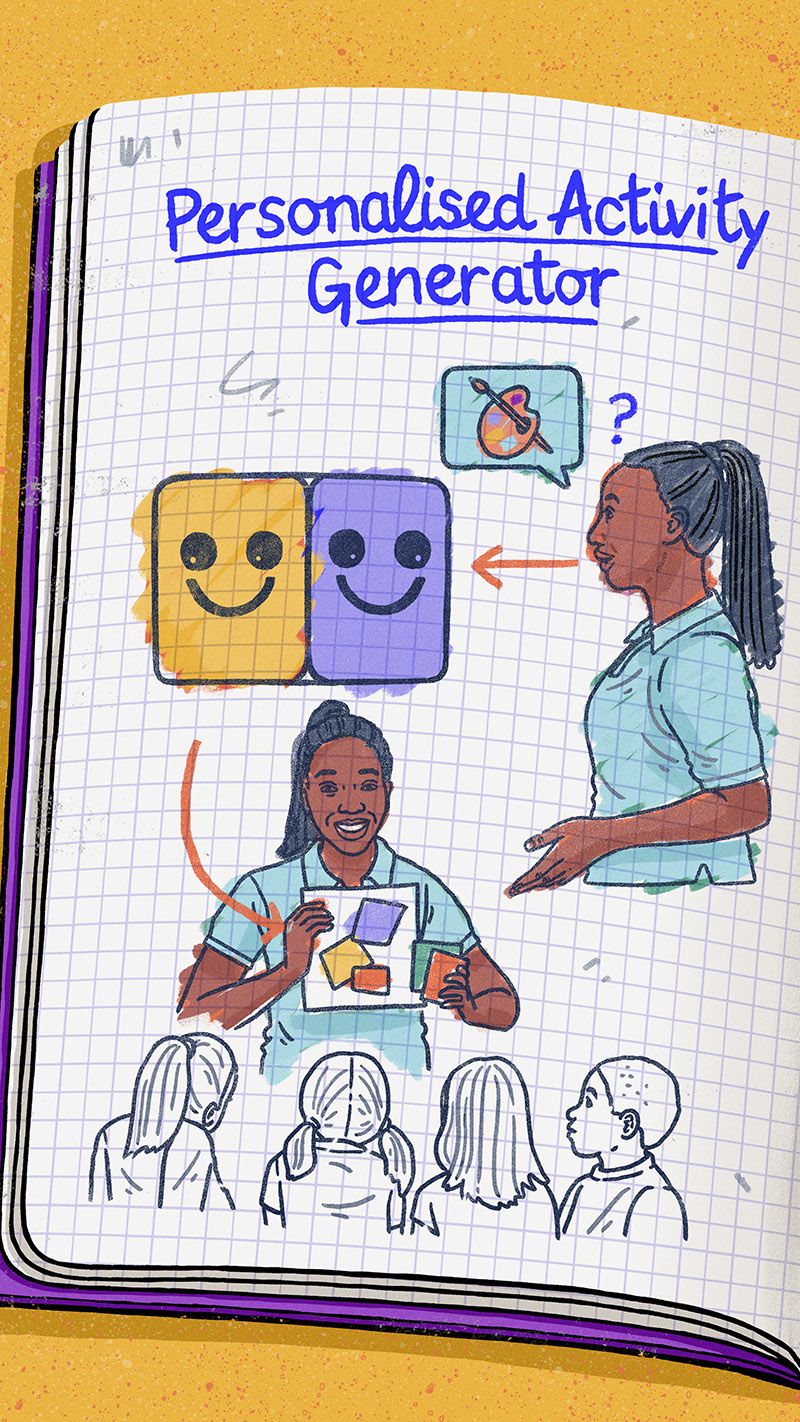
This prototype creates ideas for activities that are tailored to a child’s interests, saving time and creative energy. It refers to the Department for Education’s Development Matters guidance for England, allowing users to strengthen areas of learning that align with the curriculum for that age group.
Try it out
Imagine again that you’re in the nursery, trying to think of activities for a group of children interested in space, snails or fairies. Type in your topic and choose a predefined learning goal to get suggestions for conversations and activities.
If you describe your own learning goal, explain it in a full sentence so that the prototype can give good results.
Watch a video of this prototype in use.
Our experts’ verdict
- The prototype successfully generates a range of creative activities that combine a child’s interests with tasks from the guidance.
- Some activity descriptions are repetitive or vague, but this prototype would give a practitioner inspiration that they could build upon.
- Practitioners could become overly reliant on activity or advice generators, rather than developing their own knowledge. There is emerging evidence from other sectors that people working with AI tend to “fall asleep at the wheel” and become less skilled in their own judgement.
- The four nations of the UK use different guidelines, so tools should be adapted to work in each.
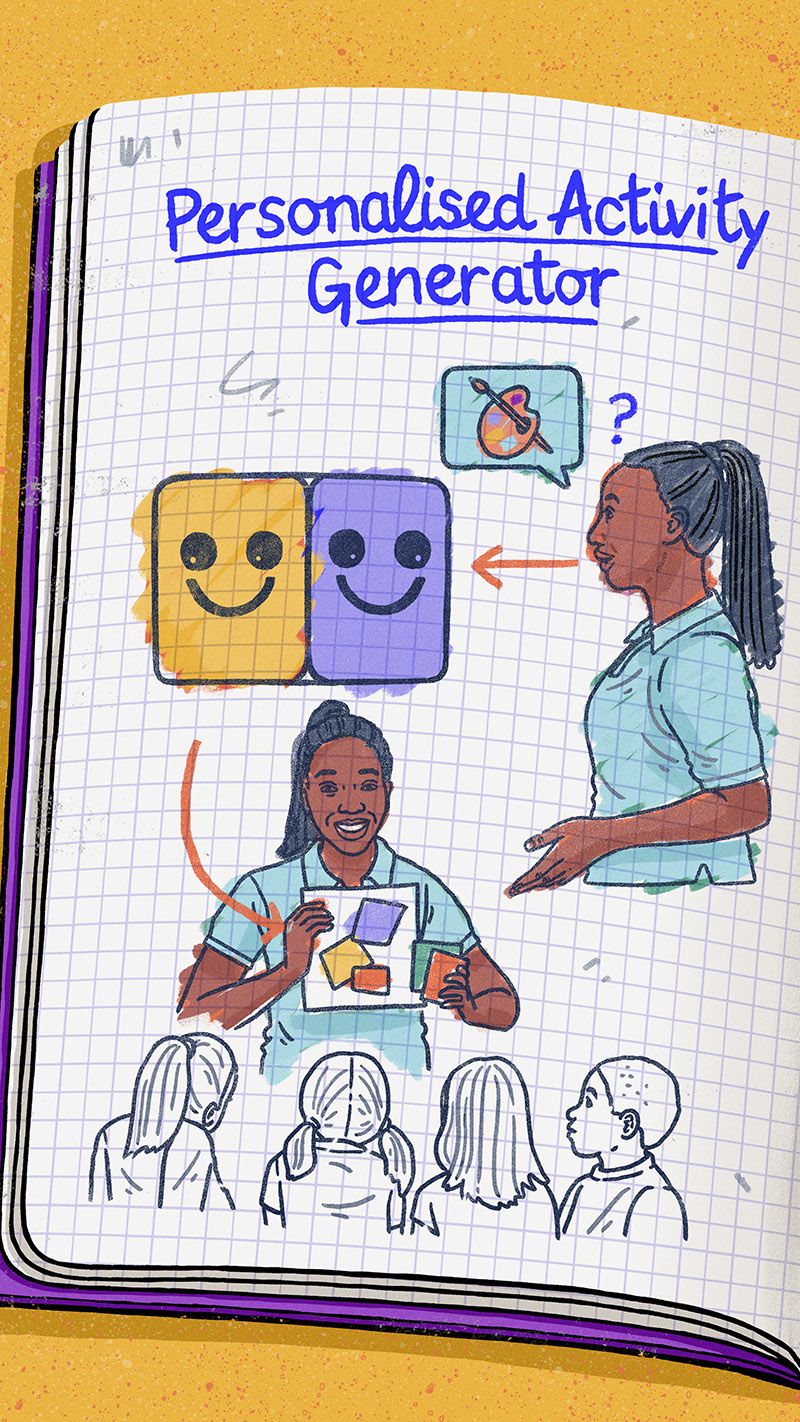
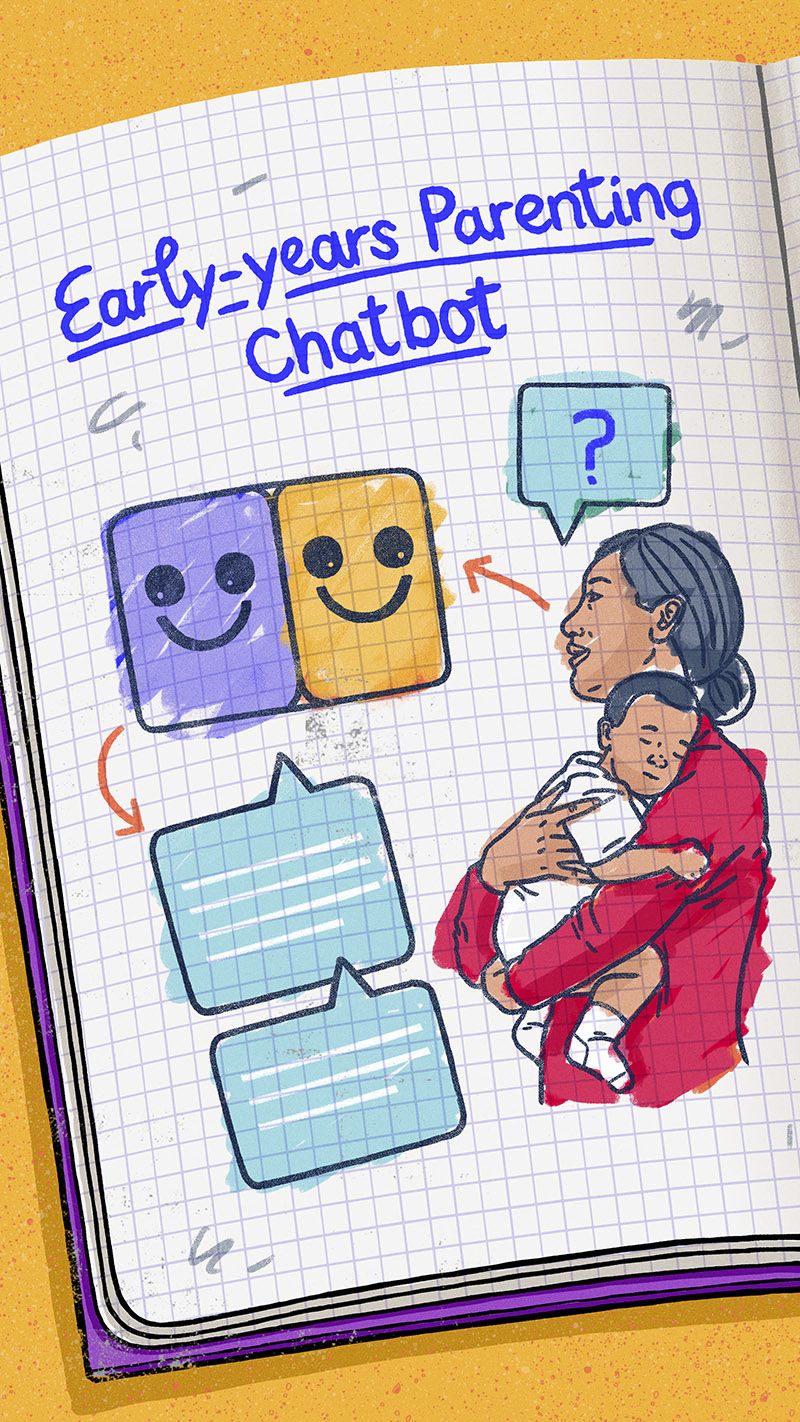
Prototype 3: Early-years parenting chatbot
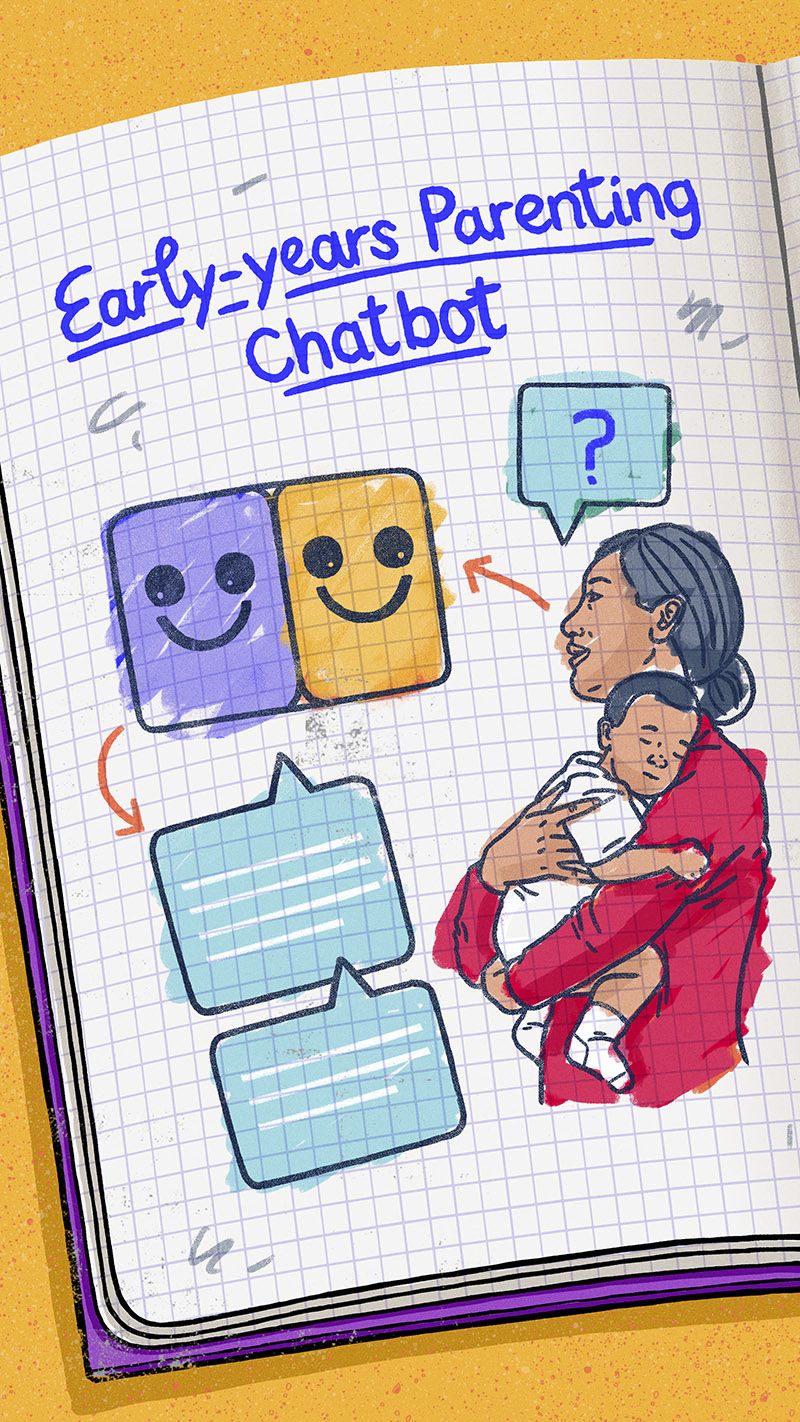
Our final prototype is a chatbot for caregivers that provides advice on various topics about babies and toddlers with an accessible and conversational tone. Accessing timely and accurate parenting advice can be challenging, with internet misinformation and limited support services hampering caregivers’ efforts to get help.
We chose the NHS Start for Life website as the basis for the chatbot’s answers because it is an openly available and trusted source of information about topics such as sleeping, feeding, and learning to speak. We also added the ability for users to leave feedback for specific responses, to help us identify problem areas for the chatbot.
Try it out
Now imagine that you are a parent of a three-year-old, dealing with a tricky situation or question you have. Your child might need calming in a doctor’s surgery, be struggling with potty training or you might want some guidance on issues with their friends.
Type your questions below. Note that this chatbot is saving the text conversations to aid our evaluation process, and you can add your feedback to the chatbot’s responses.
Watch a video of this prototype in use.
Our experts' views
- This chatbot could be very helpful to caregivers, particularly those with limited time or fewer trusted contacts.
- The advice our prototype gives is usually accurate and almost always uses a friendly and supportive tone, but it sometimes refers to information from outside the sources we’ve selected. This means it could give advice that comes from less trusted sources on the internet and it isn’t always possible to tell when this is happening.
- Privacy and the use of children's data are a concern given the lack of transparency about how many generative AI tools use the information submitted.
- Such a chatbot would need rigorous evaluation to ensure that the advice it gives is accurate and described correctly. This is a particular challenge in generative AI, where models can sometimes include information from other sources.
Evaluating the chatbot
Effective evaluation against defined criteria is crucial in developing generative AI systems, and refining evaluation methods for large language models is a significant, ongoing research area.
Guided by expert advice, we tested the chatbot’s outputs by having users compare its responses to human-written answers to parenting questions. The chatbot's responses were deemed sensible and sometimes even preferred, our initial results found. While further testing is necessary for conclusive results, these preliminary tests are beneficial for rapid prototyping, indicating the prototype's effectiveness and identifying areas for improvement.
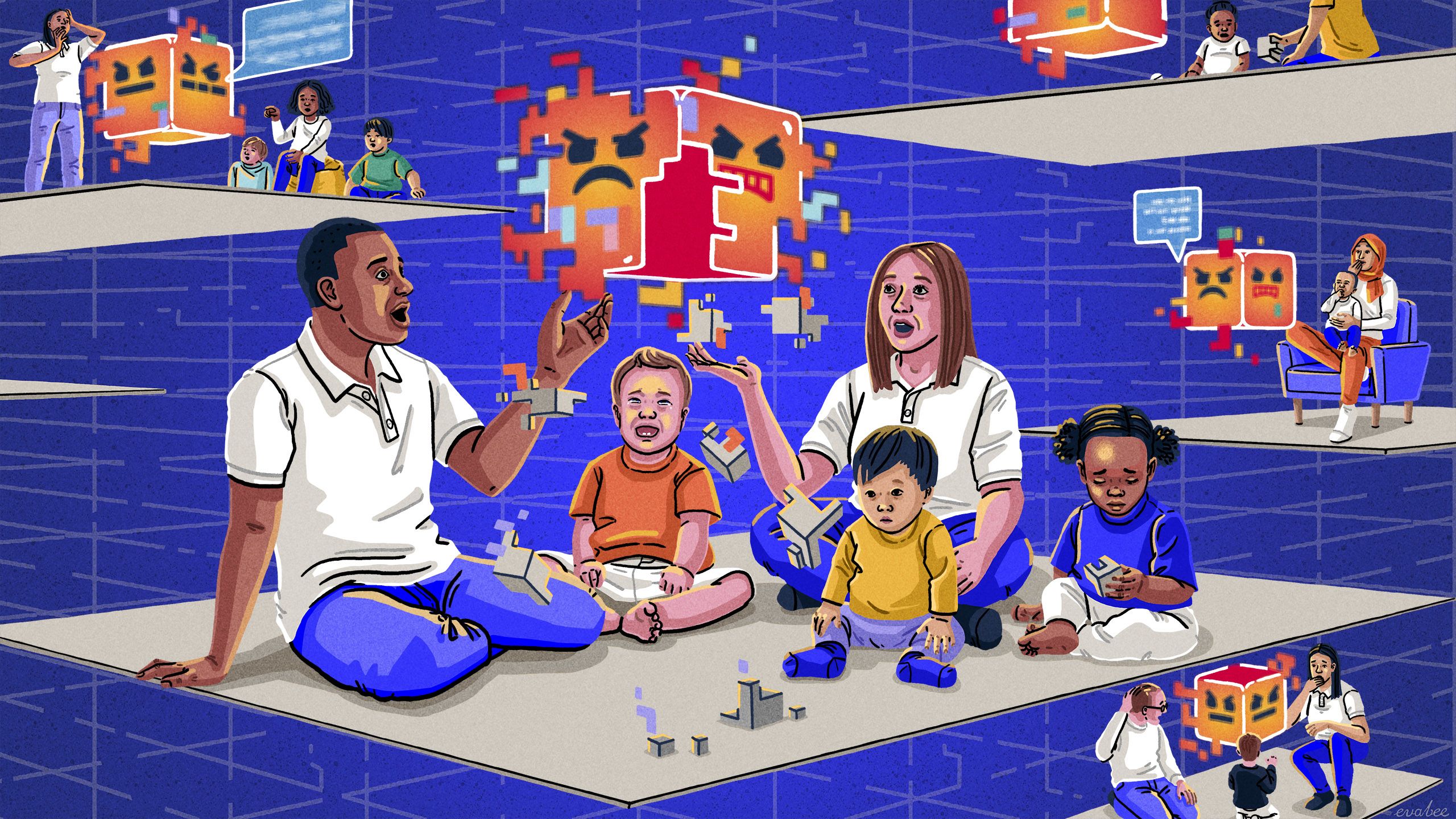
How do we avoid the pitfalls of using generative AI in early-years education?
This research suggests that generative AI has the potential to make early-years education more rewarding for practitioners and more engaging for children. There is a way to go before this new technology can have a positive impact at scale, and there are a few areas worthy of focus on the journey to getting there:
Thinking outside the box: In exploring generative AI applications, we should envision transformative changes in early-years education, not just incremental improvements. For instance, imagine a system handling all interactions with parents, employees and authorities, rather than just speeding up admin tasks. Like past technologies such as electricity, generative AI presents a chance to fundamentally rethink our approach.
Responsible experimentation: We encourage practitioners to try using generative AI tools for different tasks and publicise the results to build shared understanding across the early-years sector. This could include open sharing of algorithms, prompts and evaluation results, facilitated by trade unions and the Department for Education. Different formats should also be trialled, as in our experiments with WhatsApp.
Training and guidance: Our experts said that many early-years practitioners have lower levels of confidence with technology, so they might benefit from training to understand generative AI’s strengths and weaknesses.
Protecting children: Risks around accuracy, bias, and potential exposure to harmful content mean that young children should not use generative AI tools themselves, even under supervision. The impact of these tools on children's cognitive and social development is not fully understood and measures to filter inappropriate content have only been partially effective. Our experts were also concerned about increased screen time.
Careful procurement: Generative AI tools, being general-purpose, could become entrenched in many areas of early-years education, making switching difficult. Their widespread use also funnels more data and funding to developers, potentially centralising power and influence in a few hands. This could lead to significant influence over opinions and practices. Utilising open source tools might mitigate this concentration of power.
Systematic testing: Rigorous evaluation is essential to ensure AI tools enhance education. Such work could be accelerated by an innovation fund, similar to a previous EdTech Innovation Fund set up by the Department for Education, which helped develop better products, improved the evidence base, and grew effective use of edtech tools.
Collaborate with us: This project is the beginning of a conversation about how to make this technology work well for people working in Nesta’s mission areas. Get in touch if you’d like to collaborate with us or follow our work, and check out the Civic AI Observatory, a partnership between Nesta and Newspeak House to explore the impact of generative AI on the charity sector.
Acknowledgements
The following list of experts made valuable contributions to this work but do not necessarily support or endorse the findings.
- Adam Cantwell-Corn, Connected By Data
- Albert Phelps, Accenture
- Alexandra Powell, Cardiff Council
- Amy Greenwood, Cardiff Council
- Clare Lyons-Collins, BabyBuddy
- Clare Stead, Oliiki
- Cliff Manning, ParentZone
- Dr Caroline Chibelushi, Innovate UK
- Jen Lexmond, EasyPeasy
- Liz Hodgman, Local Government Association
- Mary Towers, TUC
- Matt Arnerich, famly
- Matt Davies, Ada Lovelace Institute
- Nick Allott, Nquiring Minds
- Nikola Nikolov, NLP Research Engineer
- Nilushka Perera, BabyBuddy
We are grateful to our colleagues in the fairer start team for their insightful reflections and feedback on the prototypes.
We thank Rosie Oxbury for her help with the chatbot evaluation process, Tom Willcocks for his help with improving the personalised activity generator prototype, and Dan Kwiatkowski and Solomon Yu for their advice regarding generative AI and large language models.
Editor: Siobhan Chan
Illustrator: Eva Bee